How about multi-task learning for virtual flow metering?
It can be extremely useful to apply multi-task learning (MTL) to the problem of virtual flow metering (VFM). Why? Because of the inherent non-stationarity of the underlying process. Part 2/5 of the MTL series.
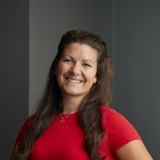
- Author
- Mathilde Hotvedt Wekre
- Publish date
- · 4 min read
In petroleum production systems, the reservoir is depleted in time. Due to this, the pressure in the reservoir decreases. This in turn causes the pressures in the rest of the production system to decline in time, for instance in each petroleum well. To compensate for the decreasing pressure, petroleum engineers typically increase the choke opening to maintain high production rates. An illustration of this is seen in Figure 1. In the figure, historical data of the choke opening and the pressure upstream the choke is visualized for 55 wells. The data points are colored for one of the wells, indicating the time since the first data point.

Imagine that you want to model a data-driven virtual flow meter solely based on historical sensor data from that well in question. After developing the model, you use it to predict the multiphase flow rates in the future - say one year ahead. What do you think the prediction accuracy would be? In general, I’d say the performance would be poor. How come, you say? Because the future states of the well have never been seen before and are not represented in the historical well data. How can you expect a data-driven model that learns only from data, to do well on data it has never seen before?
A potential fix to this issue is to learn from experiences on other wells. For example, take one chosen well from any petroleum asset. Then, if we have another petroleum well with similar characteristics that is much older than your chosen well, why not learn from the behavior of this well?
We can utilize the historical data from the old well to learn how the chosen well should behave when it ages. This is MTL in a nutshell.
But why stop with one well? Why not let all wells learn from all other wells at a petroleum asset? Or thinking even bigger, why not learn from all other wells in the world? Look at the figure below as an example. The figure illustrates the pressure distribution upstream the choke in the historical data from 55 wells. The vertical dotted lines group wells from four different assets, also indicated by color. Now, as the pressure will decline in time, the orange wells would likely benefit by learning from the green wells.

As described in the previous post, MTL differs from transfer learning by learning just one big, shared model with data from all available wells. There are two sets of parameters, one set that is common for all wells, and one set with specific parameters linked to each well. In a way, we can think of this as attempting to learn the general physical behavior of a petroleum well. Let us draw this analogy to first-principle, mechanistic simulators. In these, first-principle equations are utilized to describe the physical behavior of the process. The equations are common for all wells. But, there will be parameters that are unique for each well. For instance, the density of oil could be substantially different from one asset to another. So in a way, these two methods are not that different in the way that they try to capture both generality and specificity.
We think the concept of MTL is intriguing for the virtual flow meter application. Previous research into data-driven modeling for virtual flow metering - see several previous posts on this topic - has shown that the method is sensitive to the available data and the non-stationarity of the underlying process. In three upcoming posts in this article series (soon to be published!), we discuss the results from a recent study on MTL for VFM. For the interested, the full article may be found here. The three articles will discuss three key properties of an MTL model - robustness, making sense physically, and low maintenance.